I’ve spent quite a bit of time recently analysing clinical datasets for a couple of projects. This has reminded me of the mistakes I have made in the past, and the lessons I have learned to make life SO much easier. I thought I’d share a few thoughts!
Planning ahead and following some simple rules before collecting/entering data, can make the analysis at a later stage very much easier. When reviewing a spreadsheet of data limited to say 30 rows(e.g., Hb, platelets..… etc.), I would be fairly confident of finding errors. It takes time and patience and is not 100% accurate, but can be done. If faced however with larger databases, failure to follow these basic rules can make analysis a nightmare.
In general there are 2 types of data: numerical data and text data. Thinking first about numerical data, for clinical databases, this is often entered manually. If this is the case, there will inevitably be errors. It is essential these errors are found before any analysis begins. The importance of this cannot be overstated. I have worked on a number of projects where the resulting publication described a clinical condition. These publications may become an important reference source for clinicians diagnosing and managing the condition in question. If the clinical picture presented in the publication is wrong, then the practice of other clinicians may be influenced incorrectly with significant consequences for patients. Some examples? In a database I worked on recently, a platelet count was entered as 10.3. As many of the patients were thrombocytopenic this was not inherently wrong. However, the lab concerned reported platelet counts as integers. So this data point was clearly wrong. It may in fact have been 103, but this was impossible to confirm. So what to do? First, it is important to recognise that inclusion of this data point might have significantly affected the mean value reported. As it was a single point, this might not have been significant. However, with multiple errors this becomes more of a concern. And the clinical picture reported may be misleading. The answer is that such data points should be deleted. It is better to delete than to include a data point that is clearly wrong. Now how to find such data points? Manual checking is possible, but itself is not 100% reliable as above. Excel is often used for such databases, and there are many functions in Excel that help. Min and Max are useful here, as they will often identify outliers that should be deleted. The patient recorded as weighing 20 kg in an adult study. This will at least be identified by the min function, and a decision can then be made if this data point is correct: probably not. If the real value cannot be verified, then the data point can be deleted.
If it is necessary to be as accurate as possible double entry can be used: much like when changing your password for many computer applications. Two columns are set up for each data point, and the data entered twice. No cutting and pasting! A third column then simply uses the Excel minus ‘function’ capability to subtract one cell from the other to check if the 2 data entries agree. The answer in the third column should be 0. Any values other than 0 can be identified easily allowing data entry errors to be corrected.
Text data can be more difficult! Again, one of the most common Excel functions to use is “Countif”. This will count the number of times the specified text is entered in a given row/column of the datasheet. The problem is that if text is entered incorrectly then that data point will not be counted. I have spent many hours looking for missing data that have not been counted in such spreadsheets. Countif is not case sensitive so English and english will both be counted. But Egnlish will not. Nor will Eng-lish or Engl ish or Englis. These may look easy to identify, but in a database of perhaps 650 rows, it suddenly becomes much less straightforward. Even more difficult is that spaces at the beginning or end of words will also cause entries to be missed. These can be a nightmare to find in even quite small databases!
Even more important is planning how you will enter data. I have worked with databases with entries in a single cell such as Haemorrhage-epistaxis, Haemorrhage-GI, Haemorrhage-UGI, and Haemorrhage-LGI. If what you want to count is haemorrhage, this doesn’t work. Many will be missed. It is much better to have 2 columns with each component of the description in a separate column. They can then be counted separately. Another option is to have a numerical code, which eliminates spelling errors, but raises the possibility of incorrect ‘translation’. Thinking this through carefully before data entry starts will save much time when it comes to data analysis.
In general the message is plan ahead before starting data collection. Know how you will analyse data before you start, understand how your spreadsheet application works and enter your data in a way that makes analysis easy. Planning and forward thinking are everything!
I have really only scratched the surface of this issue here as I just wanted to flag up the importance of getting database entry right in order to make subsequent analyses as easy as possible. There is an excellent online article about Excel functions that help: Top ten ways to clear your data
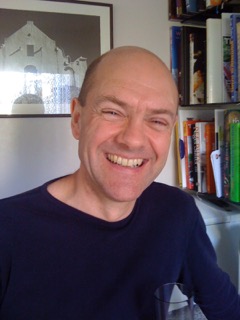